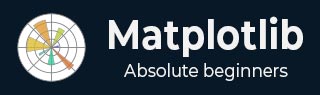
- Matplotlib Basics
- Matplotlib - Home
- Matplotlib - Introduction
- Matplotlib - Vs Seaborn
- Matplotlib - Environment Setup
- Matplotlib - Anaconda distribution
- Matplotlib - Jupyter Notebook
- Matplotlib - Pyplot API
- Matplotlib - Simple Plot
- Matplotlib - Saving Figures
- Matplotlib - Markers
- Matplotlib - Figures
- Matplotlib - Styles
- Matplotlib - Legends
- Matplotlib - Colors
- Matplotlib - Colormaps
- Matplotlib - Colormap Normalization
- Matplotlib - Choosing Colormaps
- Matplotlib - Colorbars
- Matplotlib - Text
- Matplotlib - Text properties
- Matplotlib - Subplot Titles
- Matplotlib - Images
- Matplotlib - Image Masking
- Matplotlib - Annotations
- Matplotlib - Arrows
- Matplotlib - Fonts
- Matplotlib - What are Fonts?
- Setting Font Properties Globally
- Matplotlib - Font Indexing
- Matplotlib - Font Properties
- Matplotlib - Scales
- Matplotlib - Linear and Logarthmic Scales
- Matplotlib - Symmetrical Logarithmic and Logit Scales
- Matplotlib - LaTeX
- Matplotlib - What is LaTeX?
- Matplotlib - LaTeX for Mathematical Expressions
- Matplotlib - LaTeX Text Formatting in Annotations
- Matplotlib - PostScript
- Enabling LaTex Rendering in Annotations
- Matplotlib - Mathematical Expressions
- Matplotlib - Animations
- Matplotlib - Artists
- Matplotlib - Styling with Cycler
- Matplotlib - Paths
- Matplotlib - Path Effects
- Matplotlib - Transforms
- Matplotlib - Ticks and Tick Labels
- Matplotlib - Radian Ticks
- Matplotlib - Dateticks
- Matplotlib - Tick Formatters
- Matplotlib - Tick Locators
- Matplotlib - Basic Units
- Matplotlib - Autoscaling
- Matplotlib - Reverse Axes
- Matplotlib - Logarithmic Axes
- Matplotlib - Symlog
- Matplotlib - Unit Handling
- Matplotlib - Ellipse with Units
- Matplotlib - Spines
- Matplotlib - Axis Ranges
- Matplotlib - Axis Scales
- Matplotlib - Axis Ticks
- Matplotlib - Formatting Axes
- Matplotlib - Axes Class
- Matplotlib - Twin Axes
- Matplotlib - Figure Class
- Matplotlib - Multiplots
- Matplotlib - Grids
- Matplotlib - Object-oriented Interface
- Matplotlib - PyLab module
- Matplotlib - Subplots() Function
- Matplotlib - Subplot2grid() Function
- Matplotlib - Anchored Artists
- Matplotlib - Manual Contour
- Matplotlib - Coords Report
- Matplotlib - AGG filter
- Matplotlib - Ribbon Box
- Matplotlib - Fill Spiral
- Matplotlib - Findobj Demo
- Matplotlib - Hyperlinks
- Matplotlib - Image Thumbnail
- Matplotlib - Plotting with Keywords
- Matplotlib - Create Logo
- Matplotlib - Multipage PDF
- Matplotlib - Multiprocessing
- Matplotlib - Print Stdout
- Matplotlib - Compound Path
- Matplotlib - Sankey Class
- Matplotlib - MRI with EEG
- Matplotlib - Stylesheets
- Matplotlib - Background Colors
- Matplotlib - Basemap
- Matplotlib Event Handling
- Matplotlib - Event Handling
- Matplotlib - Close Event
- Matplotlib - Mouse Move
- Matplotlib - Click Events
- Matplotlib - Scroll Event
- Matplotlib - Keypress Event
- Matplotlib - Pick Event
- Matplotlib - Looking Glass
- Matplotlib - Path Editor
- Matplotlib - Poly Editor
- Matplotlib - Timers
- Matplotlib - Viewlims
- Matplotlib - Zoom Window
- Matplotlib Plotting
- Matplotlib - Bar Graphs
- Matplotlib - Histogram
- Matplotlib - Pie Chart
- Matplotlib - Scatter Plot
- Matplotlib - Box Plot
- Matplotlib - Violin Plot
- Matplotlib - Contour Plot
- Matplotlib - 3D Plotting
- Matplotlib - 3D Contours
- Matplotlib - 3D Wireframe Plot
- Matplotlib - 3D Surface Plot
- Matplotlib - Quiver Plot
- Matplotlib Useful Resources
- Matplotlib - Quick Guide
- Matplotlib - Useful Resources
- Matplotlib - Discussion
Matplotlib - Path Effects
A path effects refer to the ways in which a path (route) can be manipulated in computer graphics. A "path" is a line or shape that you create using a drawing tool, and "path effects" allow you to apply various modifications to that line or shape.
Imagine you draw a simple line, and with path effects, you can make that line look wavy, dotted, or apply other visual changes without having to redraw it manually. It is like adding special effects to the path you have created, making your drawings more interesting and dynamic.

Path Effects in Matplotlib
You can use the "path_effects" module in matplotlib to enhance the visual representation of your plots by creating path effects. To get started, consider the "withStroke()" function within the "path_effects" module. This function allows you to add a stroke, or outline, to lines and markers.
Path effects in Matplotlib allow you to enhance the appearance of lines and shapes in your plots by applying special visual effects to them.
Simple Line with Shadow Path Effect
In Matplotlib, creating a simple line with a shadow involves drawing a basic line on a plot and then enhancing it with a shadow effect to make it visually interesting. This effect gives the appearance of a shadow under the line, as if it were creating a subtle shade on the plotting surface.
Example
In the following example, we are drawing a wavy line, and then adding shadow effect to the line using the path_effects module. The shadow effect consists of a "gray" stroke, creating the appearance of a shadow behind the line.
import matplotlib.pyplot as plt import matplotlib.patheffects as path_effects import numpy as np # Generating data x = np.linspace(0, 10, 100) y = np.sin(x) # Creating a simple line plot fig, ax = plt.subplots() line, = ax.plot(x, y, label='Simple Line') # Adding a shadow effect to the line shadow_effect = path_effects.withStroke(linewidth=5, foreground='gray') line.set_path_effects([shadow_effect]) ax.set_title('Simple Line with Shadow Effect') plt.legend() plt.show()
Output
Following is the output of the above code −

Dashed Line with Outline Path Effect
Creating a dashed line with an outline path effect in Matplotlib involves drawing a dotted line on a plot and then enhancing it by adding a bold outline. To create an outline, you can draw another line on top of the dashed line with a thicker linewidth and a solid linestyle.
Example
In here, we are creating a dashed line and enhancing it with a "black" stroke outline to give the line a bold border and make it stand out.
import matplotlib.pyplot as plt import matplotlib.patheffects as path_effects import numpy as np # Generating data x = np.linspace(0, 10, 100) y = np.cos(x) # Creating a dashed line plot with outline fig, ax = plt.subplots() line, = ax.plot(x, y, linestyle='dashed', label='Dashed Line') # Adding an outline effect to the line outline_effect = path_effects.withStroke(linewidth=3, foreground='black') line.set_path_effects([outline_effect]) ax.set_title('Dashed Line with Outline Effect') plt.legend() plt.show()
Output
On executing the above code we will get the following output −

Bold Outlined Scatter Plot Path Effect
Creating a bold outlined scatter plot with path effects in Matplotlib involves plotting a set of points on a graph and enhancing each point by adding a strong outline.
Example
In the example below, we generate a scatter with random data points. To enhance the visibility of these points, we apply a bold outline effect to each one by adding a "black" stroke with an increased "linewidth" around each scatter point.
import matplotlib.pyplot as plt import matplotlib.patheffects as path_effects import numpy as np # Generating data x = np.random.rand(50) y = np.random.rand(50) # Creating a scatter plot with bold outline effect fig, ax = plt.subplots() scatter = ax.scatter(x, y, label='Scatter Plot') # Adding a bold outline effect to the scatter points outline_effect = path_effects.withStroke(linewidth=3, foreground='black') scatter.set_path_effects([outline_effect]) ax.set_title('Scatter Plot with Bold Outline') plt.legend() plt.show()
Output
After executing the above code, we get the following output −

Combined Path Effects
Creating a combined path effects plot in Matplotlib allows us to apply multiple artistic enhancements to a line such as linestyle, markers, color gradients, and transparency.
- Linestyle − You can choose various linestyle options like solid lines ('-'), dashed lines ('--'), dotted lines (':'), and more.
- Markers − Markers can be added to highlight specific data points. Common markers include circles ('o'), squares ('s'), triangles ('^'), etc.
- Color Gradients − You can use color gradients to create visually appealing lines. You can achieve this by specifying a "colormap" and using it to color the line based on a variable.
- Transparency − Adding transparency to the line can make overlapping lines more distinguishable. You can adjust transparency using the "alpha" parameter.
Example
Now, we are applying three path effects to the line: a subtle shadow effect, a bold black outline, and a slight blur effect. The result displays a line plot with a combination of these effects.
import matplotlib.pyplot as plt import matplotlib.patheffects as path_effects import numpy as np # Generating data x = np.linspace(0, 10, 100) y = np.sin(x) # Creating a line plot fig, ax = plt.subplots() line, = ax.plot(x, y, label='Combined Effects Line') # Combine multiple path effects: Shadow, Bold Outline, and Blur shadow_effect = path_effects.withStroke(linewidth=5, foreground='cyan') outline_effect = path_effects.withStroke(linewidth=3, foreground='red') blur_effect = path_effects.withStroke(linewidth=5, foreground='magenta', alpha=0.4) # Applying the combined effects to the line line.set_path_effects([shadow_effect, outline_effect, blur_effect]) ax.set_title('Combined Path Effects Line') plt.legend() plt.show()
Output
On executing the above code we will get the following output −
