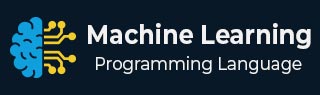
- Machine Learning Basics
- Machine Learning - Home
- Machine Learning - Getting Started
- Machine Learning - Basic Concepts
- Machine Learning - Python Libraries
- Machine Learning - Applications
- Machine Learning - Life Cycle
- Machine Learning - Required Skills
- Machine Learning - Implementation
- Machine Learning - Challenges & Common Issues
- Machine Learning - Limitations
- Machine Learning - Reallife Examples
- Machine Learning - Data Structure
- Machine Learning - Mathematics
- Machine Learning - Artificial Intelligence
- Machine Learning - Neural Networks
- Machine Learning - Deep Learning
- Machine Learning - Getting Datasets
- Machine Learning - Categorical Data
- Machine Learning - Data Loading
- Machine Learning - Data Understanding
- Machine Learning - Data Preparation
- Machine Learning - Models
- Machine Learning - Supervised
- Machine Learning - Unsupervised
- Machine Learning - Semi-supervised
- Machine Learning - Reinforcement
- Machine Learning - Supervised vs. Unsupervised
- Machine Learning Data Visualization
- Machine Learning - Data Visualization
- Machine Learning - Histograms
- Machine Learning - Density Plots
- Machine Learning - Box and Whisker Plots
- Machine Learning - Correlation Matrix Plots
- Machine Learning - Scatter Matrix Plots
- Statistics for Machine Learning
- Machine Learning - Statistics
- Machine Learning - Mean, Median, Mode
- Machine Learning - Standard Deviation
- Machine Learning - Percentiles
- Machine Learning - Data Distribution
- Machine Learning - Skewness and Kurtosis
- Machine Learning - Bias and Variance
- Machine Learning - Hypothesis
- Regression Analysis In ML
- Machine Learning - Regression Analysis
- Machine Learning - Linear Regression
- Machine Learning - Simple Linear Regression
- Machine Learning - Multiple Linear Regression
- Machine Learning - Polynomial Regression
- Classification Algorithms In ML
- Machine Learning - Classification Algorithms
- Machine Learning - Logistic Regression
- Machine Learning - K-Nearest Neighbors (KNN)
- Machine Learning - Naïve Bayes Algorithm
- Machine Learning - Decision Tree Algorithm
- Machine Learning - Support Vector Machine
- Machine Learning - Random Forest
- Machine Learning - Confusion Matrix
- Machine Learning - Stochastic Gradient Descent
- Clustering Algorithms In ML
- Machine Learning - Clustering Algorithms
- Machine Learning - Centroid-Based Clustering
- Machine Learning - K-Means Clustering
- Machine Learning - K-Medoids Clustering
- Machine Learning - Mean-Shift Clustering
- Machine Learning - Hierarchical Clustering
- Machine Learning - Density-Based Clustering
- Machine Learning - DBSCAN Clustering
- Machine Learning - OPTICS Clustering
- Machine Learning - HDBSCAN Clustering
- Machine Learning - BIRCH Clustering
- Machine Learning - Affinity Propagation
- Machine Learning - Distribution-Based Clustering
- Machine Learning - Agglomerative Clustering
- Dimensionality Reduction In ML
- Machine Learning - Dimensionality Reduction
- Machine Learning - Feature Selection
- Machine Learning - Feature Extraction
- Machine Learning - Backward Elimination
- Machine Learning - Forward Feature Construction
- Machine Learning - High Correlation Filter
- Machine Learning - Low Variance Filter
- Machine Learning - Missing Values Ratio
- Machine Learning - Principal Component Analysis
- Machine Learning Miscellaneous
- Machine Learning - Performance Metrics
- Machine Learning - Automatic Workflows
- Machine Learning - Boost Model Performance
- Machine Learning - Gradient Boosting
- Machine Learning - Bootstrap Aggregation (Bagging)
- Machine Learning - Cross Validation
- Machine Learning - AUC-ROC Curve
- Machine Learning - Grid Search
- Machine Learning - Data Scaling
- Machine Learning - Train and Test
- Machine Learning - Association Rules
- Machine Learning - Apriori Algorithm
- Machine Learning - Gaussian Discriminant Analysis
- Machine Learning - Cost Function
- Machine Learning - Bayes Theorem
- Machine Learning - Precision and Recall
- Machine Learning - Adversarial
- Machine Learning - Stacking
- Machine Learning - Epoch
- Machine Learning - Perceptron
- Machine Learning - Regularization
- Machine Learning - Overfitting
- Machine Learning - P-value
- Machine Learning - Entropy
- Machine Learning - MLOps
- Machine Learning - Data Leakage
- Machine Learning - Resources
- Machine Learning - Quick Guide
- Machine Learning - Useful Resources
- Machine Learning - Discussion
Machine Learning - Standard Deviation
Standard deviation is a measure of the amount of variation or dispersion of a set of data values around their mean. In machine learning, it is an important statistical concept that is used to describe the spread or distribution of a dataset.
Standard deviation is calculated as the square root of the variance, which is the average of the squared differences from the mean. The formula for calculating standard deviation is as follows −
$$\sigma =\sqrt{\left [\Sigma \left ( x-\mu \right )^{2}/N \right ]}$$
Where −
$\sigma$is the standard deviation
$\Sigma$ is the sum of
$x$ is the data point
$\mu$ is the mean of the dataset
$N$ is the total number of data points
In machine learning, standard deviation is used to understand the variability of a dataset and to detect outliers. For example, in finance, standard deviation is used to measure the volatility of stock prices. In image processing, standard deviation can be used to detect image noise.
Types of Examples
Example 1
In this example, we will be using the NumPy library to calculate the standard deviation −
import numpy as np data = np.array([1, 2, 3, 4, 5, 6]) std_dev = np.std(data) print('Standard deviation:', std_dev)
Output
It will produce the following output −
Standard deviation: 1.707825127659933
Example 2
Let's see another example in which we will calculate the standard deviation of each column in Iris flower dataset using Python and Pandas library −
import pandas as pd # load the iris dataset iris_df = pd.read_csv('https://archive.ics.uci.edu/ml/machine-learningdatabases/iris/iris.data', names=['sepal length', 'sepal width', 'petal length', 'petal width', 'class']) # calculate the standard deviation of each column std_devs = iris_df.std() # print the standard deviations print('Standard deviations:') print(std_devs)
In this example, we load the Iris dataset from the UCI Machine Learning Repository using Pandas' read_csv() method. We then calculate the standard deviation of each column using the std() method of the Pandas dataframe. Finally, we print the standard deviations for each column.
Output
On executing the code, you will get the following output −
Standard deviations: sepal length 0.828066 sepal width 0.433594 petal length 1.764420 petal width 0.763161 dtype: float64
This example demonstrates how standard deviation can be used to understand the variability of a dataset. In this case, we can see that the standard deviation of the 'petal length' column is much higher than that of the other columns, which suggests that this feature may be more variable and potentially more informative for classification tasks.