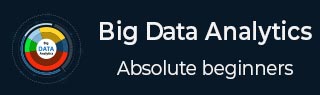
- Big Data Analytics Tutorial
- Big Data Analytics - Home
- Big Data Analytics - Overview
- Big Data Analytics - Characteristics
- Big Data Analytics - Data Life Cycle
- Big Data Analytics - Architecture
- Big Data Analytics - Methodology
- Big Data Analytics - Core Deliverables
- Big Data Adoption & Planning Considerations
- Big Data Analytics - Key Stakeholders
- Big Data Analytics - Data Analyst
- Big Data Analytics - Data Scientist
- Big Data Analytics Project
- Data Analytics - Problem Definition
- Big Data Analytics - Data Collection
- Big Data Analytics - Cleansing data
- Big Data Analytics - Summarizing
- Big Data Analytics - Data Exploration
- Big Data Analytics - Data Visualization
- Big Data Analytics Methods
- Big Data Analytics - Introduction to R
- Data Analytics - Introduction to SQL
- Big Data Analytics - Charts & Graphs
- Big Data Analytics - Data Tools
- Data Analytics - Statistical Methods
- Advanced Methods
- Machine Learning for Data Analysis
- Naive Bayes Classifier
- K-Means Clustering
- Association Rules
- Big Data Analytics - Decision Trees
- Logistic Regression
- Big Data Analytics - Time Series
- Big Data Analytics - Text Analytics
- Big Data Analytics - Online Learning
- Big Data Analytics Useful Resources
- Big Data Analytics - Quick Guide
- Big Data Analytics - Resources
- Big Data Analytics - Discussion
Big Data Analytics - Characteristics
Big Data refers to extremely large data sets that may be analyzed to reveal patterns, trends, and associations, especially relating to human behaviour and interactions.
Big Data Characteristics
The characteristics of Big Data, often summarized by the "Five V's," include −
Volume
As its name implies; volume refers to a large size of data generated and stored every second using IoT devices, social media, videos, financial transactions, and customer logs. The data generated from the devices or different sources can range from terabytes to petabytes and beyond. To manage such large quantities of data requires robust storage solutions and advanced data processing techniques. The Hadoop framework is used to store, access and process big data.
Facebook generates 4 petabytes of data per day that's a million gigabytes. All that data is stored in what is known as the Hive, which contains about 300 petabytes of data [1].

Fig: Minutes spent per day on social apps (Image source: Recode)

Fig: Engagement per user on leading social media apps in India (Image source: www.statista.com) [2]
From the above graph, we can predict how users are devoting their time to accessing different channels and transforming data, hence, data volume is becoming higher day by day.
Velocity
The speed with which data is generated, processed, and analysed. With the development and usage of IoT devices and real-time data streams, the velocity of data has expanded tremendously, demanding systems that can process data instantly to derive meaningful insights. Some high-velocity data applications are as follows −

Variety
Big Data includes different types of data like structured data (found in databases), unstructured data (like text, images, videos), and semi-structured data (like JSON and XML). This diversity requires advanced tools for data integration, storage, and analysis.
Challenges of Managing Variety in Big Data −

Variety in Big Data Applications −

Veracity
Veracity refers accuracy and trustworthiness of the data. Ensuring data quality, addressing data discrepancies, and dealing with data ambiguity are all major issues in Big Data analytics.
Value
The ability to convert large volumes of data into useful insights. Big Data's ultimate goal is to extract meaningful and actionable insights that can lead to better decision-making, new products, enhanced consumer experiences, and competitive advantages.
These qualities characterise the nature of Big Data and highlight the importance of modern tools and technologies for effective data management, processing, and analysis.