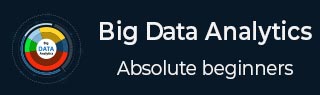
- Big Data Analytics Tutorial
- Big Data Analytics - Home
- Big Data Analytics - Overview
- Big Data Analytics - Characteristics
- Big Data Analytics - Data Life Cycle
- Big Data Analytics - Architecture
- Big Data Analytics - Methodology
- Big Data Analytics - Core Deliverables
- Big Data Adoption & Planning Considerations
- Big Data Analytics - Key Stakeholders
- Big Data Analytics - Data Analyst
- Big Data Analytics - Data Scientist
- Big Data Analytics Project
- Data Analytics - Problem Definition
- Big Data Analytics - Data Collection
- Big Data Analytics - Cleansing data
- Big Data Analytics - Summarizing
- Big Data Analytics - Data Exploration
- Big Data Analytics - Data Visualization
- Big Data Analytics Methods
- Big Data Analytics - Introduction to R
- Data Analytics - Introduction to SQL
- Big Data Analytics - Charts & Graphs
- Big Data Analytics - Data Tools
- Data Analytics - Statistical Methods
- Advanced Methods
- Machine Learning for Data Analysis
- Naive Bayes Classifier
- K-Means Clustering
- Association Rules
- Big Data Analytics - Decision Trees
- Logistic Regression
- Big Data Analytics - Time Series
- Big Data Analytics - Text Analytics
- Big Data Analytics - Online Learning
- Big Data Analytics Useful Resources
- Big Data Analytics - Quick Guide
- Big Data Analytics - Resources
- Big Data Analytics - Discussion
Big Data Adoption and Planning Considerations
Adopting big data comes with its own set of challenges and considerations, but with careful planning, organizations can maximize its benefits. Big Data initiatives should be strategic and business-driven. The adoption of big data can facilitate this change. The use of Big Data can be transformative, but it is usually innovative. Transformation activities are often low-risk and aim to improve efficiency and effectiveness.
The nature of Big Data and its analytic power consists of issues and challenges that need to be planned in the beginning. For example, the adoption of new technology makes concerns to secure that conform to existing corporate standards needs to be addressed. Issues related to tracking the provenance of a dataset from its procurement to its utilization are often new requirements for organizations. It is necessary to plan for the management of the privacy of constituents whose data is being processed or whose identity is revealed by analytical processes.
All of the aforementioned factors require that an organisation recognise and implement a set of distinct governance processes and decision frameworks to ensure that all parties involved understand the nature, consequences, and management requirements of Big Data. The approach to performing business analysis is changing with the adoption of Big Data. The Big Data analytics lifecycle is an effective solution. There are different factors to consider when we implement Big Data.
Following image depicts about big data adoption and planning considerations −

Big Data Adoption and Planning Considerations
The primary potential big data adoption and planning considerations are as −
Organization Prerequisites
Big Data frameworks are not turnkey solutions. Enterprises require data management and Big Data governance frameworks for data analysis and analytics to be useful. Effective processes are required for implementing, customising, filling, and utilising Big Data solutions.
Define Objectives
Outline your aims and objectives for implementing big data. Whether it's increasing the customer experience, optimising processes, or improving decision-making, defined objectives always give a positive direction to the decision-makers to frame strategy.
Data Procurement
The acquisition of Big Data solutions can be cost-effective, due to the availability of open-source platforms and tools, as well as the potential to leverage commodity hardware. A substantial budget may still be required to obtain external data. Most commercially relevant data will have to be purchased, which may necessitate continuing subscription expenses to ensure the delivery of updates to obtained datasets.
Infrastructure
Evaluate your current infrastructure to see if it can handle big data processing and analytics. Consider whether you need to invest in new hardware, software, or cloud-based solutions to manage the volume, velocity, and variety of data.
Data Strategy
Create a comprehensive data strategy that is aligned with your business objectives. This includes determining what sorts of data are required, where to obtain them, how to store and manage them, and how to ensure their quality and security.
Data Privacy and Security
Analytics on datasets may reveal confidential data about organisations or individuals. Analyzing different datasets includes benign data that can reveal private information when the datasets are reviewed collectively. Addressing these privacy concerns necessitates an awareness of the nature of the data being collected, as well as relevant data privacy rules and particular procedures for data tagging and anonymization. Telemetry data, such as a car's GPS record or smart metre data readings, accumulated over a long period, might expose an individual's location and behavior.

Security ensures the security of data networks and repositories using authentication and authorization mechanisms is an essential element in securing big data.

Provenance
Provenance refers to information about the data's origins and processing. Provenance information is used to determine the validity and quality of data and can also be used for auditing. It can be difficult to maintain provenance as a large size of data is collected, integrated, and processed using different phases.
Limited Realtime Support
Dashboards and other applications that require streaming data and alerts frequently require real-time or near-realtime data transmissions. Different open-source Big Data solutions and tools are batch-oriented; however, a new phase of real-time open-source technologies supports streaming data processing.
Distinct Performance Challenges
With the large amounts of data that Big Data solutions must handle, performance is frequently an issue. For example, massive datasets combined with advanced search algorithms can lead to long query times.
Distinct Governance Requirements
Big Data solutions access and generate data, which become corporate assets. A governance structure is essential to ensure that both the data and the solution environment are regulated, standardized, and evolved in a controlled way. Establish strong data governance policies to assure data quality, integrity, privacy, and compliance with legislation like GDPR and CCPA. Define data management roles and responsibilities, as well as data access, usage, and security processes.
Distinct Methodology
A mechanism will be necessary to govern the flow of data into and out of Big Data systems.

It will need to explore how to construct feedback loops so that processed data can be revised again.
Continuous Improvement
Big data initiatives are iterative, and require on-going development over time. Monitor performance indicators, get feedback, and fine-tune your strategy to ensure that you're getting the most out of your data investments.
By carefully examining and planning for these factors, organisations can successfully adopt and exploit big data to drive innovation, enhance efficiency, and gain a competitive advantage in today's data-driven world.